
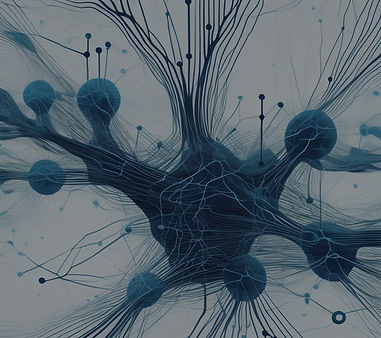
SOLUTIONS DRAWING VALUES FROM IN SILICO PREDICTIONS
Explore Our Solutions to Elevate the Value of In Silico Predictions in Decision-Making Processes
Advancing in silico predictions
In silico predictions are in a state of continuous evolution, driven by the expanding applications and persistent efforts to standardize and broaden their usage. In 2007, the OECD released guidelines pertaining to the validation of QSAR models, emphasizing the necessity for models that adhere to the following principles:
•a defined endpoint,
•an unambiguous algorithm,
•a defined domain of applicability,
•appropriate measures of goodness-of-fit, robustness, and predictivity,
•mechanistic interpretation, if possible.
Following the establishment of these guiding principles, the ICH M7 guideline was issued in 2014 for mutagenicity assessment. This marked a significant advancement in embracing in silico approaches within regulatory frameworks, where an in silico prediction was permitted as a substitute for a traditional experimental test in accordance with regulatory guidelines.
We are diligently developing in silico prediction models in alignment with the latest advancements in both scientific and regulatory domains.
ENSAMBLE MODELS
Ensemble models are machine learning approach to combine multiple other models in the prediction process. This approach offers a solution to overcome the technical challenges such as noise, bias, and variance associated with a single estimator. Hence, integrating decisions from multiple models usually enhances the overall performance.

Toxometris.ai offers Ensemble models composed of diverse conventional and cutting-edge AI/ML algorithms, including Boosting Machines, Graph Neural Networks, and Large Language Models. It is evident that various types of molecular representations embody distinct sets of information about a particular molecule. Our ensemble models applied to various types of molecular representations, including SMILES, Fingerprint, Graphs, Descriptors, extract the most information about molecule structure and properties.
RANKING SYSTEM
Every endpoint and property within the realm of ADMET predictions is undeniably crucial for evaluating potential risks associated with toxicity and drug-like characteristics. However, understanding the characteristics of a single endpoint or even multiple endpoints may not provide a comprehensive basis for decision-making. In drug discovery process or other chemicals of toxicity concern, a more holistic perspective involving the entire ADMET profile or a broader range of toxicity endpoints is necessary to fully characterize the molecule and determine the overall risk.

Toxometris.ai offers a high-throughput in silico screening platform based on a unique Risk-Score. The Risk-Score is estimated based on ADMET properties along with physicochemical and medicinal chemistry properties. This efficient approach facilitates the swift ranking of a substantial pool of compounds, delivering a list of the most and least promising candidates for acceptance as pharmaceuticals. The scoring function ranges from 0 to 1, with a lower score indicating a higher likelihood of a compound being accepted as a pharmaceutical or non-toxic chemical, and vice versa.
MECHANISTIC INTERPRETATION OF PREDICTIONS
The field of AI/ML has witnessed significant advancements over the last five years, with newer algorithms consistently outperforming traditional and simpler QSAR models. However, advanced models, while delivering superior performance, are often characterized by their black-boxes. Apart from input and output, users lack insight into the internal workings of these models. In biomedical science, where the explainability of predictions holds paramount importance the choice lies between employing simpler QSAR models with lower predictive performance but a higher level of transparency, or embracing advanced algorithms with outstanding performance but accompanied by the opacity of black boxes.

Toxometris.ai offers a powerful synergy of advanced algorithms and methodologies that enable mechanistic interpretation of results. Our predictions are grounded in the exceptional performance of ensemble models employing cutting-edge algorithms. In our comprehensive reports, users benefit from the inclusion of a read-across approach, revealing the three most similar compounds with known experimental values and mechanisms. Additionally, a rule-based approach is integrated, unveiling six structural alerts responsible for both negative and positive outcomes. This knowledge not only aids in the mechanistic interpretation of predictions but also provides valuable insights into the structural modifications needed to influence specific molecular activities.